Repairing Age-Related Mutations with Direct Meiosis Induction
Introduction
One of the main drivers of biological aging is the accumulation of genetic mutations. Many different lines of evidence support this conclusion; for example, across species, mutation rates are inversely related to lifespan, and within species, cancer incidence rates universally increase with age.1,2
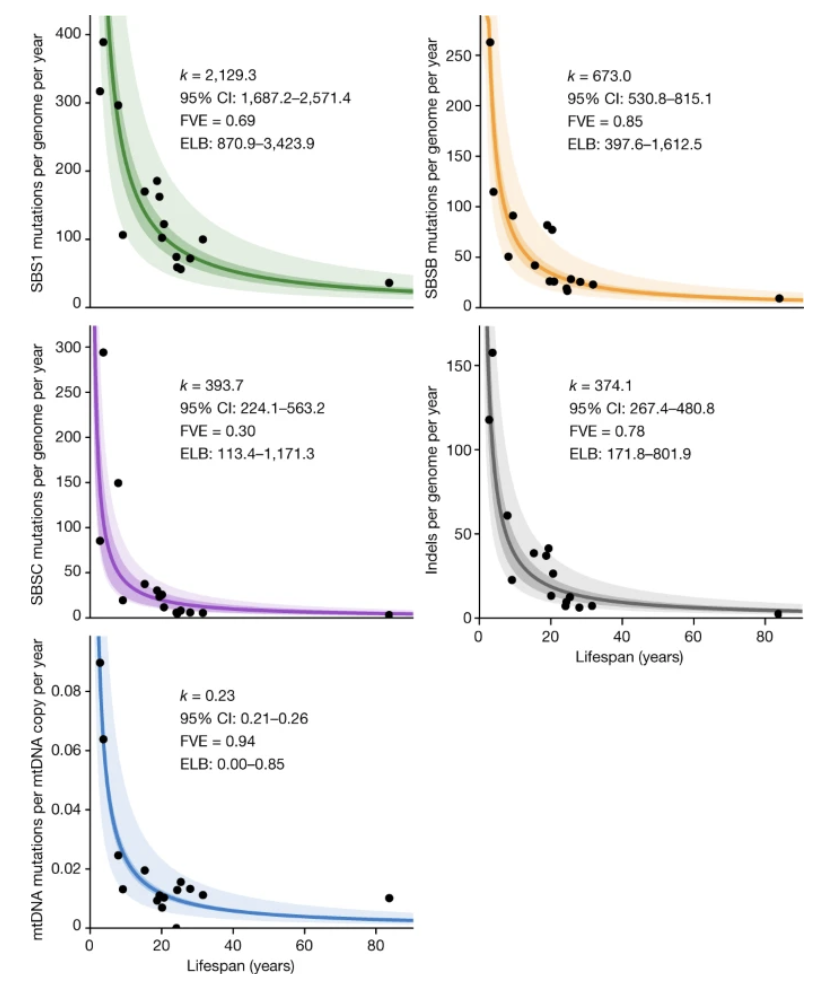
This conclusion is also intuitive: errors in genomic replication and exposure to environmental stressors accumulate over time, and evolution has little incentive to design error-correcting mechanisms that maintain genomic fidelity well past reproductive age. It naturally follows that a therapy that could “de-age” human cells, restoring them back to their original genomic state, could meaningfully ameliorate the ailments of aging and considerably prolong human lifespan.
While such therapies are out of reach with modern biotechnologies, there are several promising avenues of current research which could, in the future, form the basis of such a “de-aging” therapy. Here at Ivy Natal, we are working on one such avenue, called Direct Meiosis Induction (DMI). Although we are developing this technology for more immediate applications—namely, animal and plant breeding—we believe that the foundational research we are conducting has tremendous potential in the therapeutic arena. While speculative, we hope to highlight some potentially interesting applications of DMI in the future.
Motivation
Before diving into the details of how DMI can be used to reverse mutational accumulation, we would like to instead pose a more general question:
Suppose that you had the technology to take a cell or an organism and modify its genotype however you liked, in as many positions as you want. What would be the applications of this technology?
If we suddenly developed this capability overnight, it would be an understatement to say that it would completely revolutionize civilization. As discussed previously, restoring each individual’s cells to their “original” genotypes, free of accumulated mutations, would essentially cure the vast majority of cancers and dramatically improve overall health. The impact is, however, not limited to humans; for example, we currently know what animal and plant genotypes are most productive, but are unable to easily attain those target genotypes. Being able to instantly reach those genotypes would deliver order-of-magnitude level improvements in agricultural yields.
How close are we to achieving this technology? Unfortunately, not very. Even though the development of CRISPR-Cas9 gene editing has allowed researchers to make targeted edits to the genome, it remains monumentally challenging to “multiplex” edits, or to edit the genome precisely at a large number of different loci. These types of complex edits at hundreds or thousands of locations are precisely those with the greatest potential value, because mutations occur sporadically at random locations throughout the genome, and because complex traits are almost always highly polygenic in nature.3
Making targeted edits to the genome will always be somewhat fraught with the danger of unwanted, off-target edits that introduce potentially deleterious changes. However, we believe that Direct Meiosis Induction (DMI) offers a novel, and potentially more promising, technique: we harness the natural randomness of sexual reproduction, as well as the low cost of genomic sequencing, to repeatedly breed cells in vitro toward a desired genotype. In the context of “de-aging” human cells, we don’t even need to know what the original, un-mutated genotype is: because mutations are essentially random noise, it’s especially straightforward to remove that noise through repeated DMI, which roughly acts as a “smoothing” filter that efficiently removes sporadic noise.
What is Direct Meiosis Induction?
Let’s step back for a moment and discuss what DMI actually is. At Ivy Natal, we are reprogramming somatic cells—for example, skin cells or fat cells—into haploid cells (with one set of chromosomes) that have undergone meiosis and genetic recombination. This is analogous to trying to turn somatic cells into sperm or eggs, except with one critical difference: We believe that the genetic recombination characteristic of reproductive cells can be achieved separately from the highly complex process of phenotypic maturation into sperm or eggs. Once normal cells can be transformed into haploid cells, those haploid cells can be used to generate cell fusion via techniques such as intracytoplasmic sperm injection and somatic cell nuclear transfer, which can then be genotyped and re-differentiated into haploid cells, forming a sexual cycle entirely contained within a laboratory. In sum, we are essentially making two separate “bets”:
- 1. First, that the “hard part” of reproductive cell development is genetic recombination, and that this can be induced independently of the actual phenotypic maturation into sperm or eggs.
- 2. Second, that we can rely on cheap genotyping technology to choose which cell fusions to re-apply the process, rather than needing to actually observe their post-maturity phenotypes.
The successful development of DMI would constitute one of the purest forms of iterated selection (IS), a technology that has been theorized about for well over a decade but has yet to be realized in practice. As previously alluded to, this has typically been discussed in the context of some target genotype, such as with a cattle breeder with a highly accurate polygenic score for milk yield. Even in the absence of a particular target genotype, repeated application of DMI on cells from the same individual will rapidly reduce the incidence of unwanted somatic mutations; we will shortly see, however, that genotyping at each stage of the process considerably aids the “de-aging” process.
De-aging cells with DMI
The basic idea behind applying DMI to generate a “de-aged” pool of genotypically similar cells is to take cells from a particular individual, differentiate them into haploid cells, re-combine those haploid cells into diploid cell fusions, and repeat:
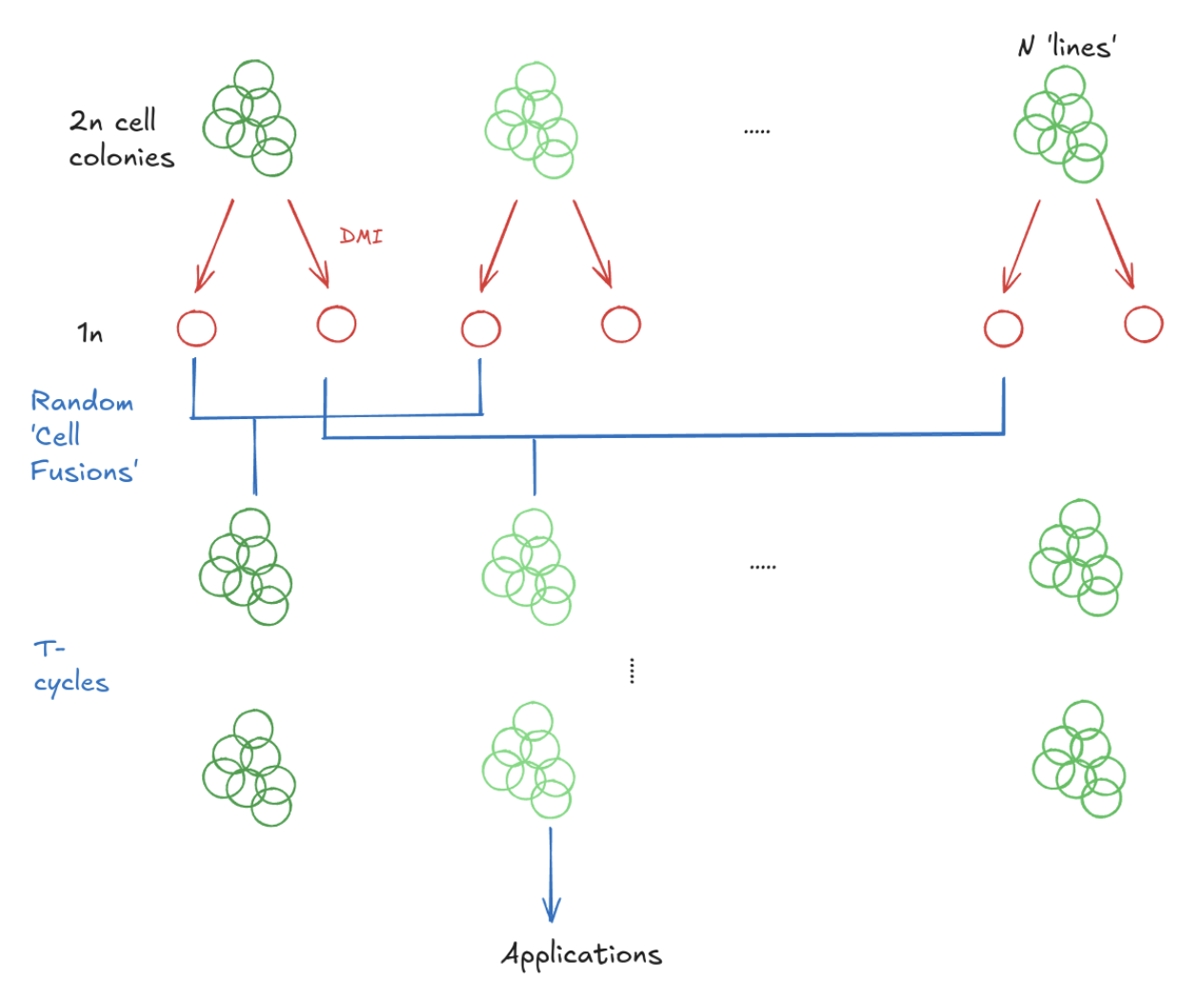
With certain simplifying assumptions, we can simulate the effectiveness of this process. Suppose, for example, that we begin with 100 initial cell “lines” (representing, e.g., 100 different biopsies taken from different sites. Under the Wright-Fisher model, where our population of cells is repeatedly re-expanded to a fixed size and randomly mating, we can see that the prevalence of somatic mutations progressively declines with the generation count:
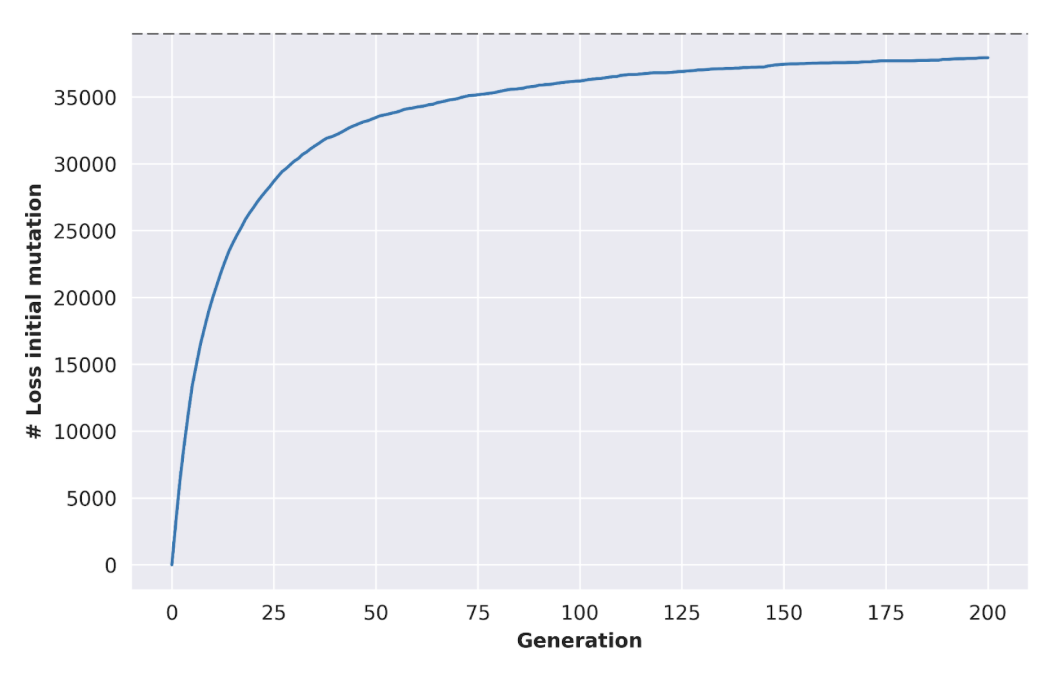
The application of DMI to “de-aging” cells has one major weakness: namely, cells will acquire new mutations as they are progressively cultured, directly diminishing the benefits of DMI. In the absence of any additional competing processes that actively select away cell fusions with higher mutational load, the introduction of de novo mutations renders DMI much less effective:
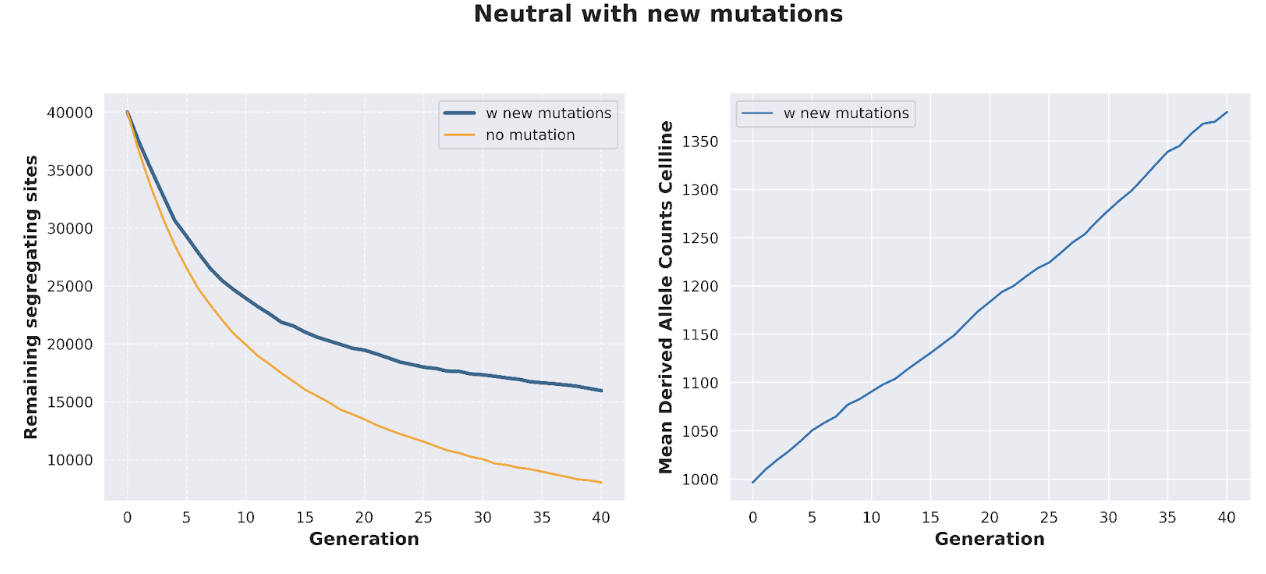
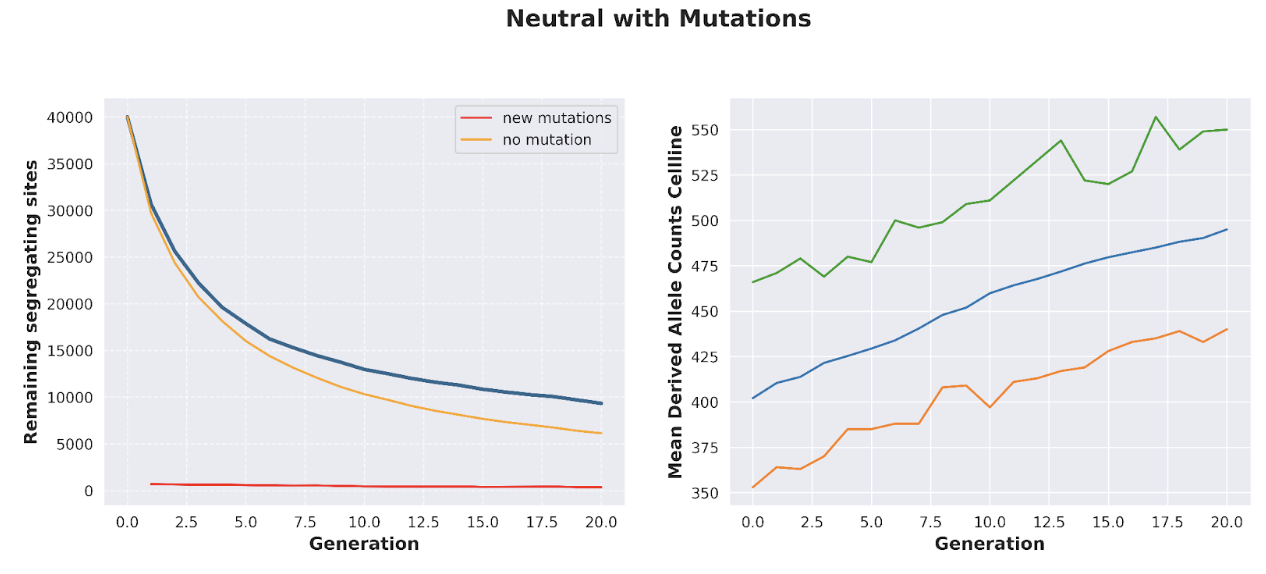
Additionally, mutations that favor survival in vitro will be favored; while this is not necessarily always a negative factor (for example, novel mutations that are too deleterious will not actually survive on to the next generation), there are many cases, such as with tumor suppressor p53, where overactivation of certain genes aids proliferation in culture but results in enhanced tumor risk in vivo.
Thankfully, as we previously alluded to, we can address these shortcomings by introducing additional genomic selection at each somatic recombination stage: once haploid cells are recombined into diploid cell fusions, each cell fusion can be genotyped, and only a certain subset of those cell fusions are chosen to progress to the next iteration.
The most simple way to select cell fusions at each generation is to calculate the number of somatic mutations for each cell fusion and only allow the top k to progress (expanding them out sufficiently to keep population size constant). In practice, this empirically results in a rate of decline for somatic mutations that resembles a power law curve: perhaps a familiar sight to evolutionary biologists as a common outcome whenever deterministic (selection) and stochastic (mutation) forces are commingled together:
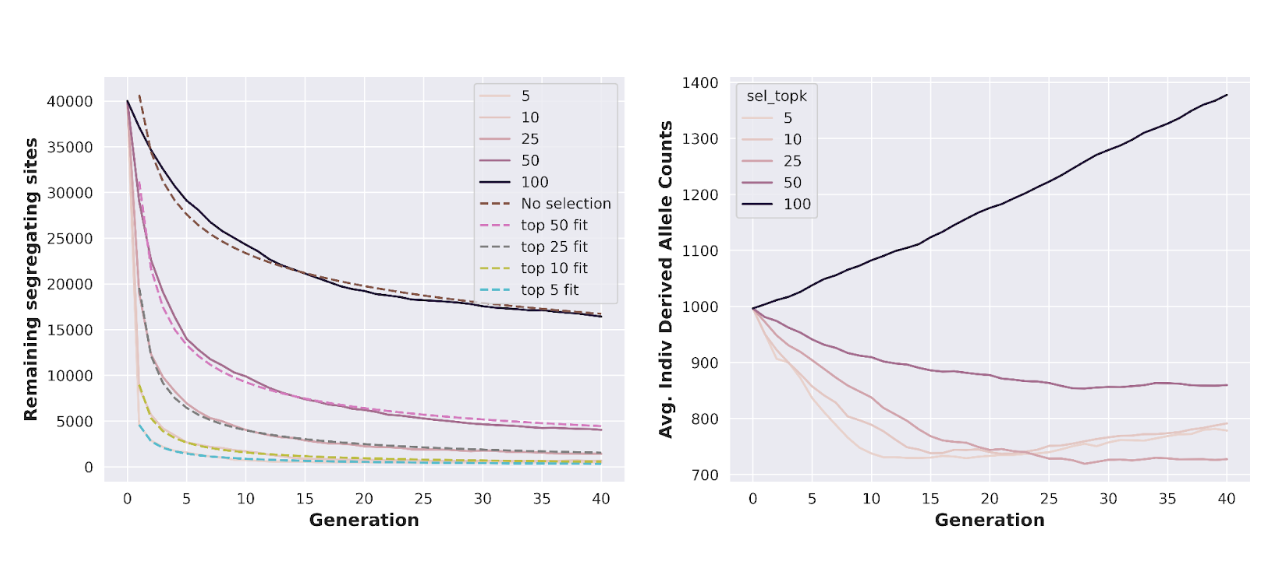
The strategy we are implementing here—genotyping diploid cell fusions, assigning a very crude score to each one, and selecting the top k cell fusions at each generation—admits many possibilities for improvements. For example, a certain degree of backcrossing can yield somewhat modest improvements in the rate of mutation loss; more interestingly, genotyping and selection can be performed at the haploid level, leading to the construction of extremely complex breeding strategies that optimize the pairing of haploid cells at each generation to very rapidly achieve the target genotype. These strategies quickly become too complex to discuss fully in this post, although we have simulated several to verify their basic viability.
Interestingly, the development of these optimal breeding strategies remains, by and large, an open question, with the state of the art methods applying reinforcement learning in a somewhat “brute force” approach. At Ivy Natal, we are building up a deep reservoir of ML-native talent and internal bioinformatics tooling that we anticipate will give us a substantial competitive advantage in the application of DMI to agricultural breeding.
Let’s take a step back and look at what we have demonstrated here: that the iterated breeding process enabled by DMI allows, even under relatively conservative assumptions, for the rapid “de-aging” of an individual’s cellular material. While the resulting cells will not be genetically identical to the source individual (e.g., at heterozygous sites), they will be largely free of the hundreds of deleterious somatic mutations that individual has accumulated over the course of their lifespan. This allogeneic population of cells could serve any number of therapeutic purposes: for example, they could be re-differentiated into target tissues for use in transplantation or be used as the starting point for CAR-T therapies. More importantly, this is a technology that might actually exist in several years’ time—everything that we have described so far is entirely possible in principle, with the barriers to full realization growing thinner and thinner with each passing year.
Closing notes
The astute reader will notice there is much we have yet to discuss. For example, how do we address the potential for deleterious runs of homozygosity in the derived cell line? Can we really assume that the derived lines are allogeneic and won’t provoke an immune response? Is it truly necessary to achieve this through a complex breeding process—or is it conceivable that multiplexed CRISPR-Cas9 will get us here in a decade or two? How else could this technology be applied in practice?
We hope to answer at least some of these questions in future posts.
References
- 1. Cagan, A. et al. Somatic mutation rates scale with lifespan across mammals. Nature 604, 517–524 (2022).
- 2. Knudson, A. G. Mutation and cancer: statistical study of retinoblastoma. Proc. Natl. Acad. Sci. U. S. A. 68, 820–823 (1971).
- 3. Boyle, E. A., Li, Y. I. & Pritchard, J. K. An Expanded View of Complex Traits: From Polygenic to Omnigenic. Cell 169, 1177–1186 (2017).